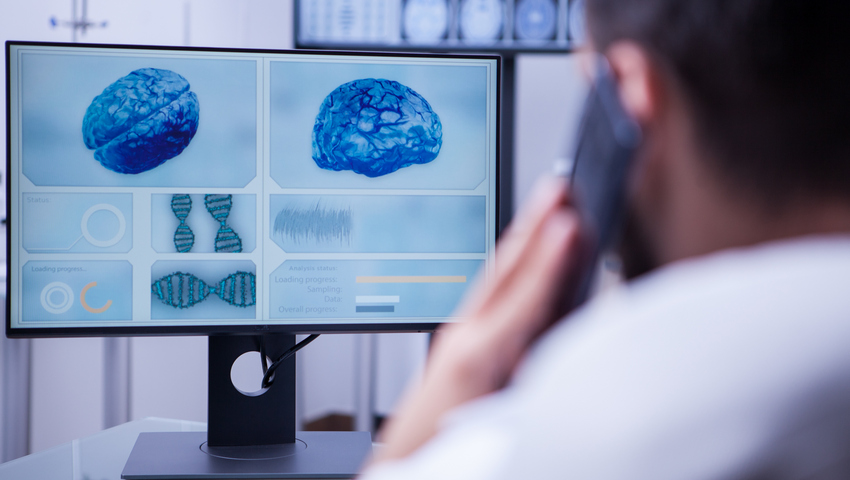
AI model enhances cancer origin identification for precise treatment
Researchers from MIT and the Dana-Farber Cancer Institute have devised a novel method to address the challenge of identifying the origin of cancer in a subset of patients. This challenge arises when physicians are unable to pinpoint the source of cancer, making it difficult to determine the most suitable treatment, as many cancer drugs are tailored to specific cancer types.
The newly developed approach leverages machine learning and involves the creation of a computational model. This model can analyze the genetic sequence of around 400 genes and utilize this information to predict the source of a particular tumor within the body.
Through this method, the team successfully managed to accurately classify over 40 percent of tumors with an unknown origin in a dataset containing approximately 900 patients. This breakthrough allowed for a remarkable 2.2-fold increase in the number of patients who could potentially be candidates for personalized, genomically guided treatments, based on the identified source of their cancer.
Intae Moon, lead author of the study and a graduate student in electrical engineering and computer science at MIT, emphasized the significant finding that the model could potentially aid medical professionals in making treatment decisions by guiding them toward personalized therapies for patients with cancers of unknown primary origin.
Alexander Gusev, senior author of the paper and an associate professor of medicine at Harvard Medical School and the Dana-Farber Cancer Institute, highlighted the impact of this work, particularly on individuals with cancers of unknown primary origin, a condition affecting around 3 to 5 percent of cancer patients.
Traditionally, the lack of knowledge regarding the primary site of cancer origin has impeded doctors from administering targeted treatments. These treatments, tailored to specific cancer types, are often more effective and have fewer side effects than generalized treatments prescribed for a broad spectrum of cancers.
The study's methodology centered on analyzing routinely collected genetic data from Dana-Farber. The data encompassed genetic sequences of approximately 400 genes commonly mutated in cancer. The researchers trained a machine-learning model using data from nearly 30 000 patients with 22 known cancer types. Subsequently, this model, named OncoNPC, was tested on around 7 000 previously unseen tumors with known origins. It demonstrated an accuracy rate of roughly 80 percent, which rose to approximately 95 percent for high-confidence predictions.
Upon these promising outcomes, the model was applied to a dataset of roughly 900 tumors from individuals with cancers of unknown primary origin. The model successfully generated high-confidence predictions for 40 percent of these cases.
The model's predictions were further validated by comparing them with the analysis of germline mutations in a subset of tumors. The model's predictions are often aligned with the cancer type predicted by these genetic mutations. Moreover, the model's predictions were aligned with patients' survival times and their responses to treatments.
By enabling the identification of the cancer's source, the researchers effectively expanded the pool of patients who could benefit from targeted treatments that were already available. The research was supported by various foundations, including the National Institutes of Health and the Louis B. Mayer Foundation.
Moving forward, the researchers aim to enhance their model by incorporating additional types of data, such as pathology and radiology images, to provide a more comprehensive prediction encompassing various data modalities. This could enable the model not only to predict tumor types and patient outcomes but potentially also recommend optimal treatment strategies.
Machine learning techniques have frequently been employed by scientists to detect diseases, showcasing their versatility and potential in the field. The Qudata team has further illuminated the indispensable role of AI and machine learning in healthcare through various projects. Our team has made significant strides in advancing medical diagnostics, particularly in the domain of cervical spine fracture detection. Our innovative solution intricately combines advanced image processing methodologies and machine learning models to discern cervical spine fractures from CT scans. This pioneering approach, grounded in the analysis of bone structure and density, empowers precise localization of fracture sites. As a result, medical professionals can swiftly and accurately diagnose fractures, preempting potential complications and optimizing patient care.
For an in-depth exploration of Qudata's endeavors and achievements read QuData AI/ML Case Studies, which shed further light on the groundbreaking work conducted by our team.