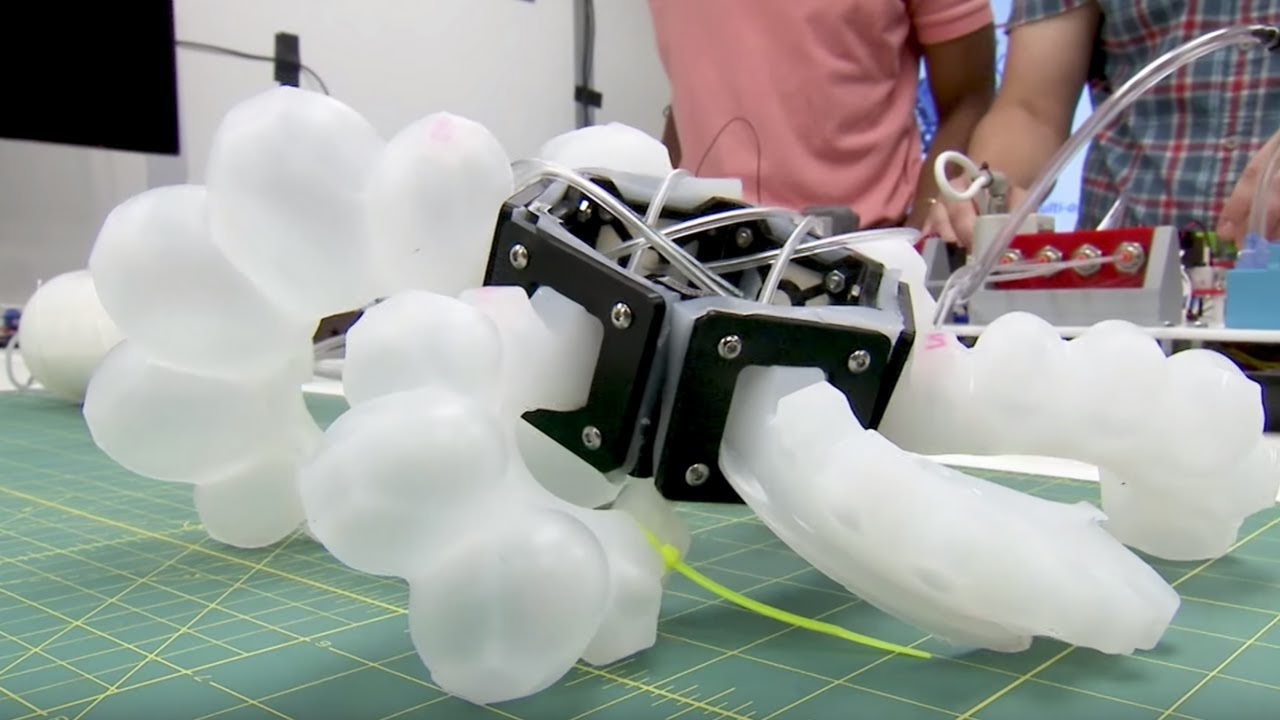
Researchers' Algorithm for Soft Robots
Deep-learning Formula for Robots
MIT scientists have actually created a formula to assist engineers design soft robotics that collect more useful details concerning their environments. The deep-learning formula suggests an enhanced positioning of sensors within the robot's body, enabling it to better communicate with its setting and complete designated jobs. The development is a step toward the automation of robotic design. "The system not only discovers a provided task, however likewise just how to best style the robot to fix that task," claims Alexander Amini. "Sensing unit positioning is a really difficult problem to resolve. So, having this option is incredibly exciting."
The study will certainly exist throughout April's IEEE International Seminar on Soft Robotics as well as will be published in the journal IEEE Robotics and also Automation Letters. Co-lead writers are Amini and also Andrew Spielberg, both PhD pupils in MIT Computer Science and Artificial Intelligence Research Laboratory (CSAIL). Various other co-authors consist of MIT PhD student Lillian Chin, and also professors Wojciech Matusik and Daniela Rus.
Creating soft robots that complete real-world jobs has been a long-running difficulty in robotics. Their stiff counterparts have an integrated advantage: a limited variety of activity. Rigid robotics' finite selection of joints as well as limbs typically produces convenient estimations by the algorithms that control mapping as well as movement planning. Soft robots are not so tractable.
Adaptable Soft-bodied Robotics
Soft-bodied robotics are adaptable and pliant - they usually feel a lot more like a lively sphere than a bowling round. "The major problem with soft robotics is that they are infinitely dimensional," says Spielberg. "Any type of point on a soft-bodied robotic can, theoretically, flaw by any means possible." That inconveniences making a soft robotic that can map the location of its body components. Past efforts have actually used an exterior camera to chart the robot's position and feed that information back right into the robot's control program. However the researchers intended to create a soft robotic untethered from exterior help.
"You can't put an infinite variety of sensing units on the robot itself," claims Spielberg. "So, the question is: The amount of sensing units do you have, as well as where do you place those sensors so as to get the most bang for your buck?" The group looked too deep for an answer.
Semantic Network
The researchers developed an unique semantic network style that both maximizes sensor placement and discovers to effectively complete jobs. Initially, the scientists split the robot's body into regions called "particles." Each particle's rate of strain was offered as an input to the semantic network. Via a process of trial and error, the network "discovers" one of the most effective sequences of movements to finish tasks, like gripping objects of different dimensions. At the same time, the network tracks which fragments are made use of most often, as well as it chooses the lesser-used fragments from the set of inputs for the networks' succeeding trials.
By maximizing the most crucial particles, the network likewise suggests where sensors need to be positioned on the robotic to guarantee reliable performance. For example, in a substitute robot with a grasping hand, the formula could recommend that sensors be concentrated in and around the fingers, where specifically controlled communications with the setting are crucial to the robot's ability to manipulate objects. While that may appear evident, it turns out the algorithm significantly outmatched people' intuition on where to cite the sensing units.
The researchers pitted their formula versus a collection of expert forecasts. For three various soft robot layouts, the group asked roboticists to by hand choose where sensors must be placed to enable the effective conclusion of jobs like grasping numerous objects. After that they ran simulations contrasting the human-sensorized robotics to the algorithm-sensorized robots. As well as the outcomes weren't close. "Our design vastly outmatched humans for each and every task, even though I looked at several of the robot bodies and also felt really confident on where the sensing units must go," claims Amini. "It turns out there are a great deal even more subtleties in this problem than we initially anticipated."
Automated Process
Spielberg states their job can aid to automate the process of robotic style. Along with creating formulas to manage a robot's motions, "we likewise need to think of exactly how we're mosting likely to sensorize these robotics, and exactly how that will certainly interact with other elements of that system," he claims. As well as far better sensor placement could have commercial applications, especially where robots are utilized for fine jobs like grasping. "That's something where you need an extremely robust, well-optimized sense of touch," states Spielberg. "So, there's a possibility for immediate impact."
"Automating the style of sensorized soft robots is an essential action towards quickly producing intelligent devices that help people with physical tasks," says Rus. "The sensing units are a crucial element of the process, as they allow the soft robot to "see" as well as recognize the world and also its partnership with the globe."
This research was funded, in part, by the National Scientific Research Foundation as well as the Fannie and also John Hertz Structure.