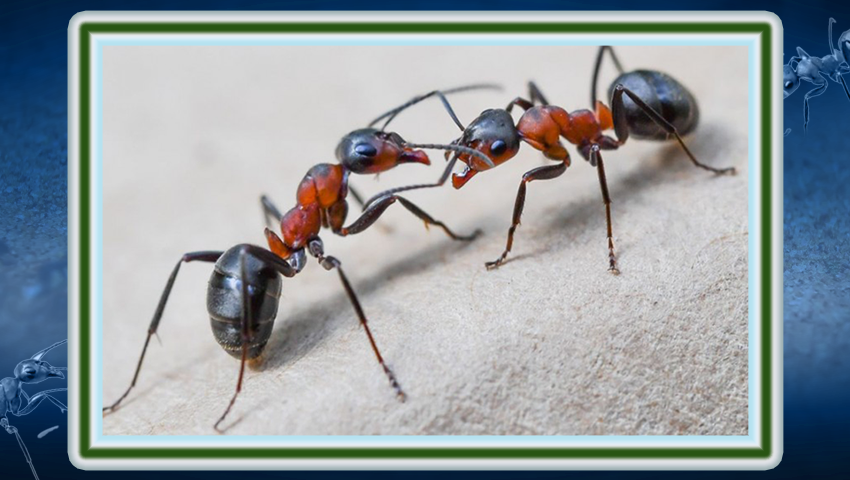
Ant-Inspired Neural Network Boosts Robot Navigation
In a rapidly evolving era of artificial intelligence (AI), the integration of AI into agriculture is taking center stage. Among the latest innovations, the Ecorobotix, a seven-foot-wide GPS-assisted solar-powered unit, elegantly glides through crop fields, targeting and eradicating weeds with an astounding 95% accuracy, effectively reducing waste. Additionally, Energid, Universal Robots are radically changing citrus fruit harvesting through a combination of multiple cameras and flexible robotic arms. The River LettuceBot employs crop geometry scanning to optimize growth and minimize pesticide usage, distinguishing between weeds and crops to prevent oversaturation and diseases.
However, the current challenge lies in navigating complex, ever-changing natural environments, such as dense forests or tall grass fields. How can robots effectively remember where they've been and recognize places they've visited before in visually repetitive surroundings?
Inspiration was found in an unlikely source: ants. These tiny creatures exhibit remarkable navigational skills despite their relatively simple sensory and neural systems. Researchers, led by Le Zhu at the Universities of Edinburgh and Sheffield, sought to mimic the navigational prowess of ants in a new artificial neural network. This network would assist robots in recognizing and remembering routes in intricate natural environments, especially in agriculture, where dense vegetation poses a significant challenge.
Ants employ a unique neural structure known as "mushroom bodies" in their brains to detect visual patterns and store spatiotemporal memories, allowing them to navigate visually repetitive surroundings effectively. Zhu and his team used this biological mechanism as inspiration for their research.
Their approach involved designing a bioinspired event camera mounted on a terrestrial robot to capture visual sequences along routes in natural outdoor environments. To facilitate route recognition, they developed a neural algorithm for spatiotemporal memory that closely mirrors the insect mushroom body circuit.
Crucially, they employed neuromorphic computing, emulating the structure and function of biological neurons, to encode memory in a spiking neural network running on a low-power neuromorphic computer. The result was a robotic system that could evaluate visual familiarity in real-time from event camera footage, supporting route recognition for visual navigation.
In rigorous testing across different settings, including grasslands, woodlands, and farmlands, the ant-inspired neural model proved its effectiveness. It outperformed another route learning method called SeqSLAM when evaluated on repeated runs on the same route or routes with small lateral offsets. SeqSLAM is a technique that matches sequences of images to find similarities between different runs.
The implications of this research extend far beyond robotics. This ant-inspired neural model holds the promise of transforming agricultural robotics, making it more efficient and effective in navigating through dense vegetation. Furthermore, researchers suggest that this model's principles could be extended to other sensory modalities, such as olfaction or sound, enhancing a robot's perception of its environment.
This study represents a significant step forward in harnessing the collective wisdom of nature's navigators to enhance our technological advancements. As we continue to draw inspiration from the natural world, AI-driven robotics could find even more innovative solutions to complex challenges, ultimately benefiting industries far and wide.
Read more breaking news about robot navigation here.