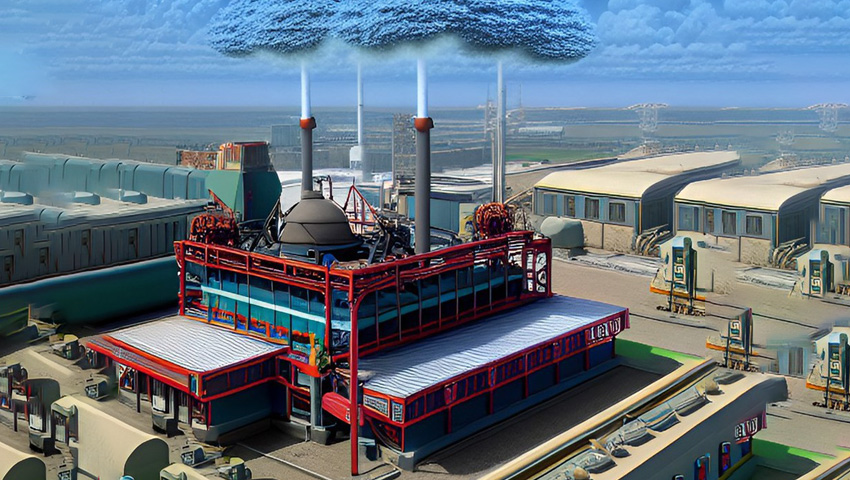
Application of machine learning to forecast amine emissions
One of the main causes of climate change on our planet is an excess of carbon dioxide emissions. It occurs predominantly in electricity generation and industrial processes, including steel and cement manufacturing. Currently, engineers and chemists are looking into ways to capture carbon that could sequester and store carbon dioxide, thereby preventing it from being released into the atmosphere.
The concept of carbon capture is an effective way to reduce greenhouse gas emissions. Special carbon capture plants work on the basis of amine technology, using amines - chemical compounds that can dissolve carbon dioxide. Amines are also used in many industries, such as pharmaceuticals, production of epoxy resins and dyes.
The problem is that amines can be potentially harmful to the environment as well as human health, so it is critical to mitigate their impact. When using amines in carbon capture plants, emissions also need to be controlled, which is a challenge due to the technological difference between the plants.
A team of scientists from EPFL's School of Basic Sciences and The Research Centre for Carbon Solutions of Heriot-Watt University has developed a new solution based on machine learning to predict amine emissions from carbon capture plants. The solution was experimentally tested in a real plant in Germany led by a team with Professor Berend Smit from EPFL's School of Basic Sciences and Professor Susana Garcia from The Research Centre for Carbon Solutions of Heriot-Watt University in Scotland as the heads of the research.
The experiments were conducted at the largest coal-fired power plant in Germany, where the next-generation amine solution has been tested at a pilot plant for more than a year. However, it has been found that amines can be released with flue gas. This presented a problem since amine emissions must be controlled.
Professor Susana Garcia, together with the owner of the plant, RWE, and TNO in the Netherlands, developed a stress test to study amine emissions under various process conditions. Professor Garcia described that they designed an experimental campaign to understand how and when amine emissions would be generated. But some of the experiments also required the intervention of plant operators to ensure its safe operation.
These interventions led to the question of how to interpret the data. Are the amine releases the result of the stress tests themselves, or has operator interference indirectly affected the emissions? This has been further complicated by a general misunderstanding of the scientific mechanisms underlying amine releases.
"In short, we had an expensive and successful campaign that showed that amine emissions can be a problem, but no tools to further analyze the data," reports Smit.
It really looked like an unsolvable problem. All measurements were taken every five minutes and a lot of data was collected. And then Kevin Maik Jablonka decided to use machine learning for pattern recognition to predict future amine emissions based on plant data. By using a new amine emission prediction model, the researchers were able to separate emissions caused by operator intervention from emissions caused by the stress test.
The model showed that some interventions had the opposite effect on emissions of solvent components. Thus, the mitigation strategies required for capture facilities operating on a single component solvent (e.g., monoethanolamine) need to be reviewed if they are operated using a mixture of amines.
"I am very enthusiastic about the potential impact of this work; it is a completely new way of looking at a complex chemical process," says Smit. "This type of forecasting is not something one can do with any of the conventional approaches, so it may change the way we operate chemical plants."