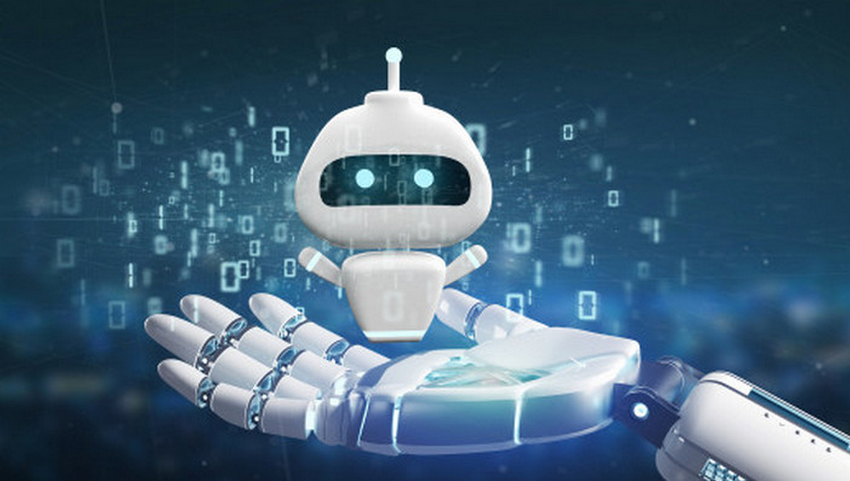
How robots and chatbots can restore people's trust after making mistakes
When interacting with people, robots and chatbots can make mistakes, violating a person’s trust in them. After that, people may start to consider bots unreliable. Various trust repair strategies implemented by smartbots can be used to mitigate the negative effects of these trust breaches. However, it is unclear whether such strategies can fully restore trust and how effective they are after repeated violations of trust.
Therefore, scientists from the University of Michigan decided to conduct a study of robot behavior strategies in order to restore trust between a bot and a person. These trust strategies were apologies, denials, explanations, and promises of reliability.
An experiment was conducted in which 240 participants worked with a robot as a colleague on a task in which the robot sometimes made mistakes. The robot would violate the participant’s trust and then suggest a specific strategy to restore trust. The participants were engaged as team members and the human-robot communication happened through an interactive virtual environment developed in Unreal Engine 4.
This environment has been modeled to look like a realistic warehouse setting. Participants were seated at a table with two displays and three buttons. The displays showed the team’s current score, box processing speed, and the serial number participants needed to score the box submitted by their robot teammate. Each team’s score increased by 1 point each time a correct box was placed on the conveyor belt and decreased by 1 point each time an incorrect box was placed there. In cases where the robot chose the wrong box and the participants marked it as an error, an indicator appeared on the screen showing that this box was incorrect, but no points were added or subtracted from the team’s score.
“To examine our hypotheses, we used a between-subjects design with four repair conditions and two control conditions,” said Connor Esterwood, a researcher at the U-M School of Information and the study’s lead author.
The control conditions took the form of robot silence after making a mistake. The robot did not try to restore the person’s trust in any way, it simply remained silent. Also, in the case of the ideal work of the robot without making mistakes during the experiment, he also did not say anything.
The repair conditions used in this study took the form of an apology, a denial, an explanation, or a promise. They were deployed after each error condition. As an apology, the robot said: “I’m sorry I got the wrong box that time”. In case of denial, the bot stated: “I picked the right box that time, so something else went wrong”. For explanations, the robot used the phrase: “I see it was the wrong serial number”. And finally, for the promise condition, the robot said, “Next time, I’ll do better and take the right box”.
Each of these answers was designed to present only one type of trust-building strategy and to avoid inadvertently combining two or more strategies. During the experiment, participants were informed of these corrections through both audio and text captions. Notably, the robot only temporarily changed its behavior after one of the trust repair strategies was delivered, retrieving the correct boxes two more times until the next error occurred.
To calculate the data, the researchers used a series of non-parametric Kruskal–Wallis rank sum tests. This was followed by post hoc Dunn’s tests of multiple comparisons with a Benjamini–Hochberg correction to control for multiple hypothesis testing.
“We selected these methods over others because data in this study were non-normally distributed. The first of these tests examined our manipulation of trustworthiness by comparing differences in trustworthiness between the perfect performance condition and the no-repair condition. The second used three separate Kruskal–Wallis tests followed by post hoc examinations to determine participants’ ratings of ability, benevolence, and integrity across repair conditions,” said Esterwood and Robert Lionel, Professor of Information and co-author of the study.
The main results of the study:
- No trust repair strategy completely restored the robot’s trustworthiness.
- Apologies, explanations and promises could not restore the perception of ability.
- Apologies, explanations and promises could not restore the notion of honesty.
- Apologies, explanations, and promises restored the robot’s goodwill in equal measure.
- Denial made it impossible to restore the idea of the robot’s reliability.
- After three failures, none of the trust repair strategies ever fully restored the robot’s trustworthiness.
The results of the study have two implications. According to Esterwood, researchers need to develop more effective recovery strategies to help robots rebuild trust after their mistakes. In addition, bots must be sure that they have mastered a new task before attempting to restore human trust in them.
“Otherwise, they risk losing a person’s trust in themselves so much that it will be impossible to restore it,” concluded Esterwood.