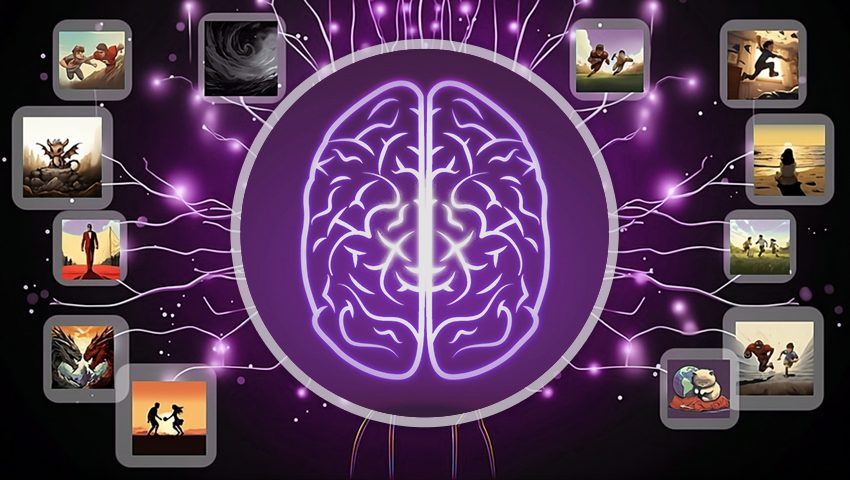
StableRep: transforming how AI learns
In a stride toward more efficient and unbiased machine learning, researchers at MIT have introduced a game-changing model StableRep. This innovative system leverages synthetic images to enhance the efficiency of artificial intelligence training.
StableRep transcends traditional methods by generating synthetic images through text-to-image transformation models, such as Stable Diffusion. It goes beyond pixels, creating entire worlds with the power of words.
At the heart of StableRep is the strategy known as "multi-positive contrastive learning." Instead of merely feeding data into the model, StableRep teaches it high-level concepts through context and variation. By treating multiple images from the same text prompt as different views of the same thing, StableRep helps the model understand the true meaning behind the images.
The groundbreaking use of synthetic images has yielded impressive results, surpassing the performance of even top-tier models trained on real images, such as SimCLR and CLIP. This breakthrough not only tackles the challenges of data collection in machine learning but also propels us into a new era of AI training methods.
Historically, data collection has been a cumbersome process, from photographing information in the 1990s to manually searching for data on the internet in the 2000s. However, raw, unfiltered data often carries biases, distorting the AI model's perception of reality. With its ability to create diverse synthetic images on command, StableRep offers an efficient solution that can significantly reduce the costs and resources associated with data collection.
A crucial element of StableRep's success is the fine-tuning of the "guidance scale" in the generative model. This delicate adjustment balances how synthetic images differ from each other while adhering to the original concept. After precise tuning, synthetic images prove to be as effective, if not more so, than their real counterparts in training self-supervised models.
Taking a bold step forward, language supervision is introduced, leading to the creation of StableRep+. Trained on 20 million synthetic images, StableRep+ not only achieves exceptional accuracy but also demonstrates remarkable efficiency compared to models trained on a staggering 50 million real images.
However, challenges lie ahead. The researchers openly acknowledge that image generation with StableRep is a slow process. Discrepancies may exist between text prompts and images. Model biases may be amplified. Furthermore, determining the authorship or ownership of an image may prove challenging. Resolving these issues is crucial for future advancements.
The reduced dependence of the StableRep model on large sets of real images raises concerns among researchers about hidden biases in the data used for text-to-image transformation models. The choice of text prompts, integral to the image synthesis process, is not entirely free from potential bias, emphasizing the need for careful text selection and human oversight.
In the words of Lijie Fan, MIT Ph.D. student and lead researcher, "Our work signifies a step forward in visual learning, offering cost-effective training alternatives while highlighting the need for ongoing improvements in data quality and synthesis."
Overall, StableRep has demonstrated a significant impact on the AI community. David Fleet, a researcher from Google DeepMind and the University of Toronto, sees this as evidence that we are nearing the dream of creating useful data for AI training. It provides compelling proof that contrastive learning based on massive datasets of synthetic images can outperform real data on a large scale, promising improvements in AI performance in the future.
MIT's StableRep is not just a breakthrough; it's a transformative force paving the way to a better future in AI training. We are entering an era where the importance of continual improvement in data quality and synthesis is impossible to overstate.