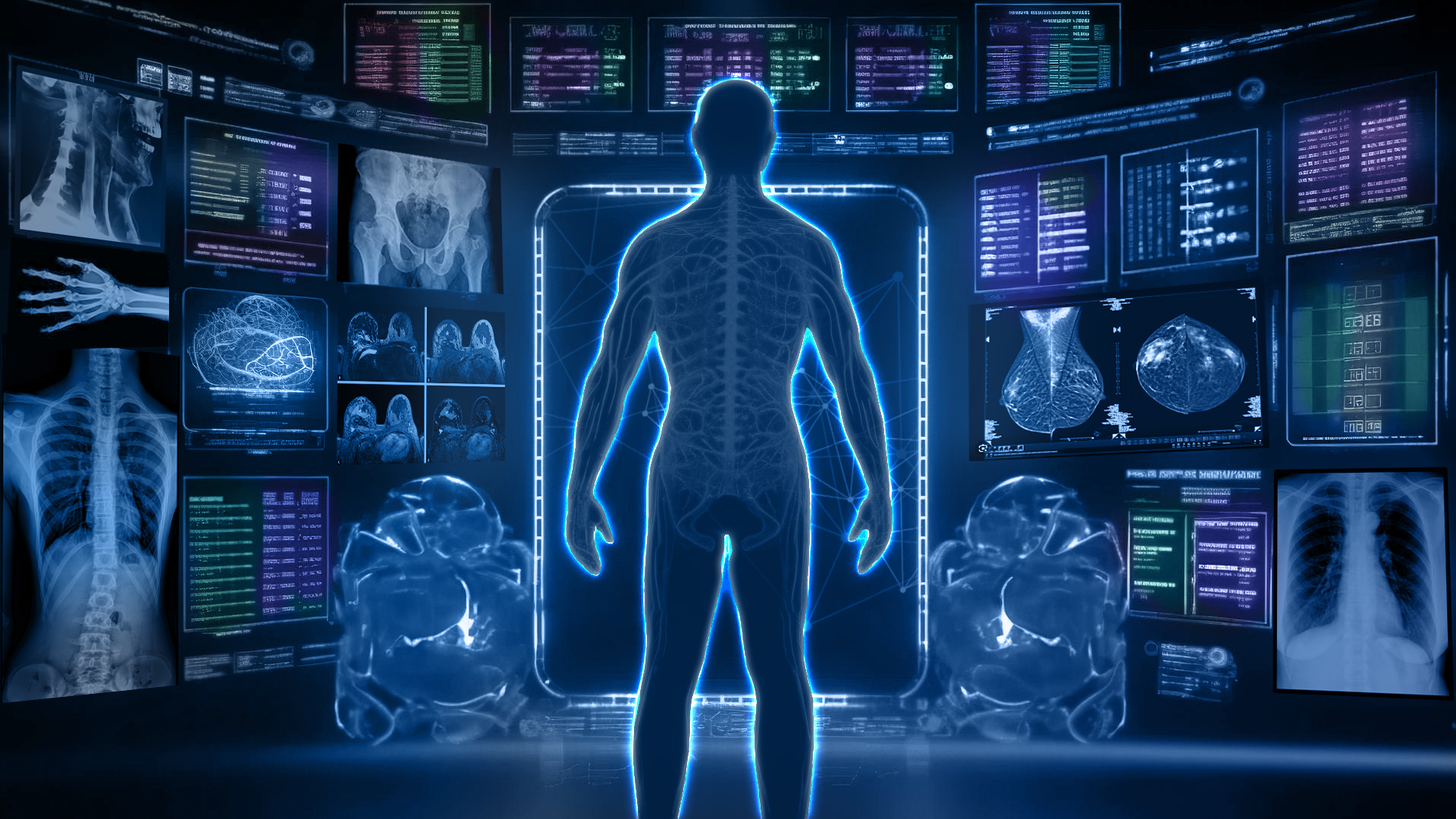
The rise of AI in radiology: how AI is reshaping medical imaging
In recent decades, artificial intelligence (AI) has gained immense importance in various fields, and medicine is no exception. The use of AI in radiology introduces new possibilities for precise and rapid diagnostics, which can significantly improve treatment outcomes and increase the efficiency of medical institutions.
Radiology is a branch of medicine that deals with the diagnosis and treatment of various diseases using medical images such as X-rays, CT scans, magnetic resonance imaging and others. Radiology is one of the most sought-after and dynamic specialties in modern healthcare, as it allows physicians to quickly and accurately determine the causes, stages and spread of diseases, as well as monitor treatment effectiveness.
However, the field of radiology also faces challenges that medical institutions and specialists encounter.
- Large volume and complexity of medical images. With the advancement of medical equipment and technologies, the volume of medical images generated in radiological examinations has significantly increased. This creates vast amounts of data, the analysis and interpretation of which require high qualifications, attention, and time.
- Shortage of qualified radiologists. With the increasing volume and complexity of medical images, there is a shortage of qualified radiologists capable of conducting diagnostics and interpreting data. This is particularly relevant for regions with low access to healthcare or for specialties requiring a high level of expertise, such as cancer diagnosis.
In these conditions, artificial intelligence represents a powerful and promising tool that can help address these problems and improve the quality and efficiency of radiology.
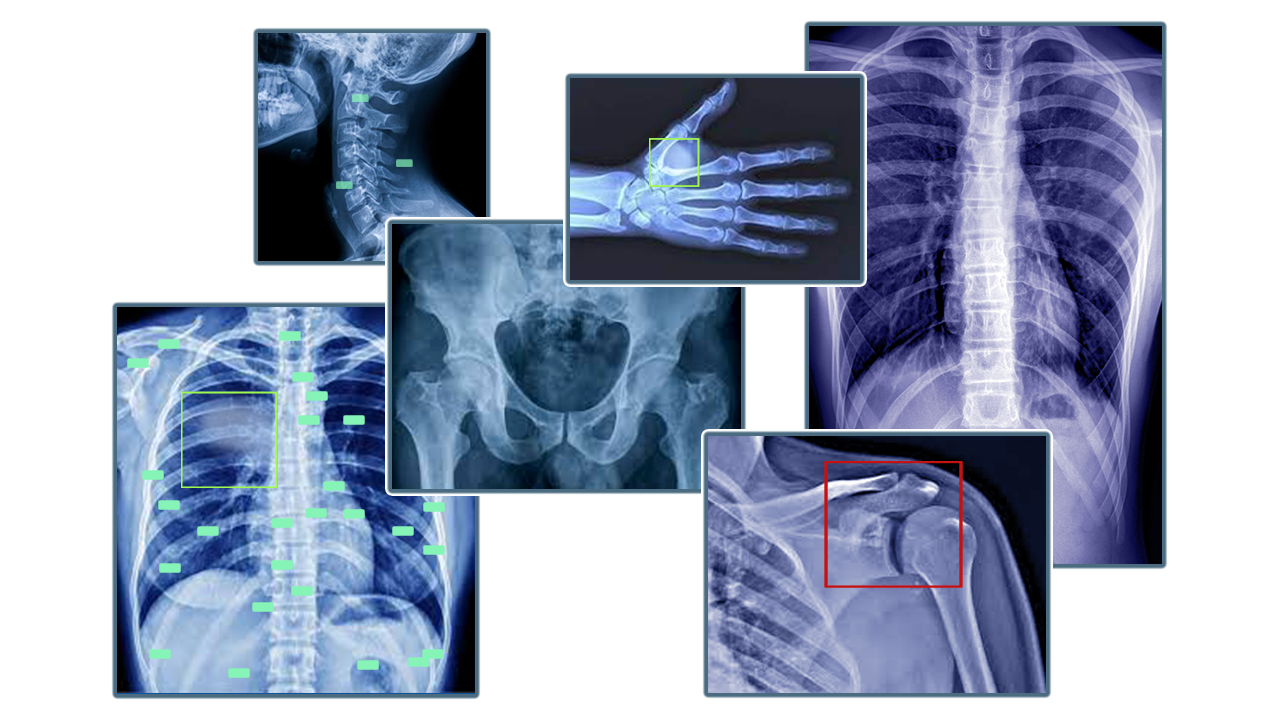
Artificial intelligence in radiology
The application of artificial intelligence in radiology is very broad and diverse, as it can assist in various stages and aspects of the radiological process.
1. Preparation and preprocessing of medical images. This includes quality enhancement, segmentation, and annotation of images.
2. Analysis and interpretation of medical images. This involves the detection, classification, localization and characterization of anomalies, pathologies and biomarkers in images.
3. Decision support and recommendations. It means assisting in diagnosis, prognosis, risk assessment, and treatment courses based on medical images and other clinical data.
4. Automation and optimization of workflows and resources. It carries out planning, distribution, prioritization, archiving, and transmission of medical images and related data.
Various methods and technologies of AI are used to achieve these tasks.
- Computer vision provides the analysis and interpretation of visual information from medical images. It is used for automatic recognition of structures and textures in images, separating bones, organs, and vessels. Computer vision is also used to create 3D models of anatomical structures based on 2D images. This allows for better visualization of complex anatomical structures and treatment planning.
- Machine learning (ML) enables systems to learn from big datasets and improve their performance over time. ML can be used for automatic segmentation of organs and tissues, allowing areas of interest to be identified in images. ML algorithms can also predict the likelihood of developing a certain disease or assess the effectiveness of a specific treatment.
- Deep learning, a subset of machine learning, uses multi-layer neural networks to process complex and unstructured data. This helps to accurately detect pathologies and anomalies in medical images: tumors, cysts, deformations, or injuries. Deep learning can also identify individual characteristics that can influence treatment choices, such as predicting responses to certain medications or therapeutic methods.
- Natural language processing (NLP) specializes in the analysis and understanding of text records from medical reports and recommendations. This allows for extracting useful information about patients' health conditions and automating documentation processes.
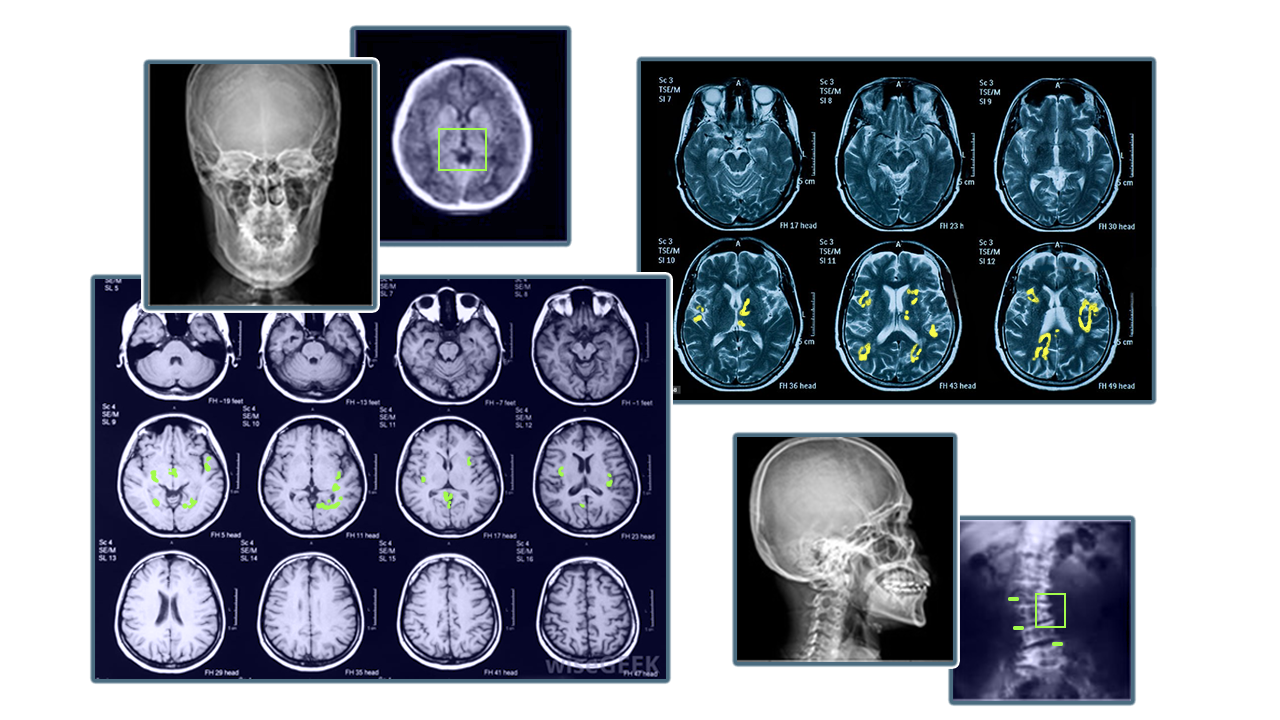
The main advantages of AI in radiology
The methods and technologies discussed above make a significant contribution to improving the efficiency of radiology departments and enhancing patient treatment outcomes. In this context, it is important to consider the main advantages of using AI in radiology.
Automation and acceleration of diagnostics
One of the key benefits of using AI in radiology is the ability to automate and speed up the process of diagnosing medical images. This includes not only detecting pathologies but also organ segmentation, anomaly identification, and disease classification. Automating these processes reduces the time spent by radiologists analyzing images, thereby increasing the productivity and efficiency of radiology departments.
Improved diagnostic accuracy
Another important advantage of AI in radiology is the improvement of diagnostic accuracy. For example, deep learning algorithms can detect even the subtlest signs of pathologies in medical images, helping to identify diseases at earlier stages of development. More accurate diagnosis allows for earlier treatment initiation and increases the chances of a successful treatment course for patients.
Personalized treatment
One more significant aspect of using AI in radiology is its ability to provide personalized solutions for each patient. Developed algorithms can analyze medical data and predict individual disease risks, enabling physicians to develop optimal treatment and health monitoring strategies. This personalized approach helps improve treatment outcomes and reduce the risk of complications.
Enhanced productivity and resource optimization
Automating processes using AI allows for increased productivity in radiology departments and optimization of resource usage. This reduces the time spent on diagnostics and increases the efficiency of medical institutions. As a result, funds are also freed up for acquiring new equipment and investing in new technologies.
Development of medical innovations
The application of AI in radiology stimulates innovative medical development, contributing to the creation of new diagnostic and treatment methods. The development of new algorithms and technologies in machine learning opens up new possibilities for improving healthcare and enhancing the quality of life for patients.
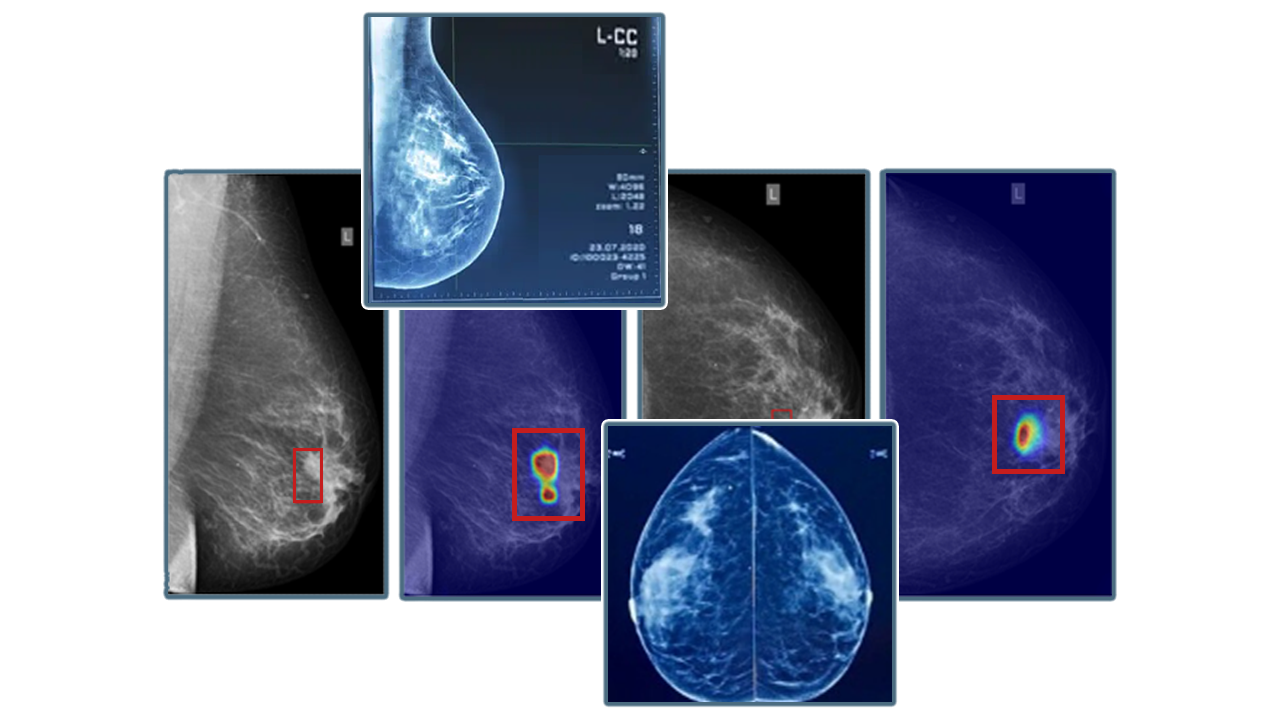
Use cases of AI in radiology and medical image analysis
- Detection and diagnosis of oncological diseases: breast cancer, lung cancer, skin cancer, prostate cancer, and others. AI can assist in detecting early signs and microscopic changes in medical images, as well as determining the type, stage, and aggressiveness of tumors.
Efficient breast cancer computer-aided detection from QuData already helps identify oncopathology at early stages, increases the accuracy of breast cancer diagnosis, reduces the likelihood of missed diagnoses, and false-positive results. Sign up for the QuData console to try the AI-driven solution for free.
- AI can determine the presence, type, and severity of bone fractures. Based on bone density data, it also assists in assessing the risk of osteoporosis, which is one of the causes of fractures.
Learn more about advanced image processing methods and machine learning models for detecting cervical spine fractures based on computed tomography data in the article The future of spine trauma diagnosis: advanced AI techniques for fracture detection".
- AI enables the diagnosis of various eye diseases such as glaucoma, diabetic retinopathy, age-related macular degeneration, cataracts, and others.
Read more about the achievements of AI in detecting retinal diseases at the cellular level in our case study AI-aided analysis of retinal fluorescent biomarker".
The use of artificial intelligence in radiology speeds up the process of analyzing medical images. Automation and improved diagnostic accuracy help radiologists work more efficiently and enhance the quality of medical services for patients. With the development of machine learning technologies and expanded access to data, the use of AI in radiology will continue to evolve, opening up new possibilities for medical diagnostics and treatment.
Iryna Tkachenko, marketing manager
References:
1. How artificial intelligence is driving changes in radiology
2. Natural language processing for breast imaging: a systematic review
3. Workforce crisis in radiology in the UK and the strategies to deal with it: is artificial intelligence the saviour?